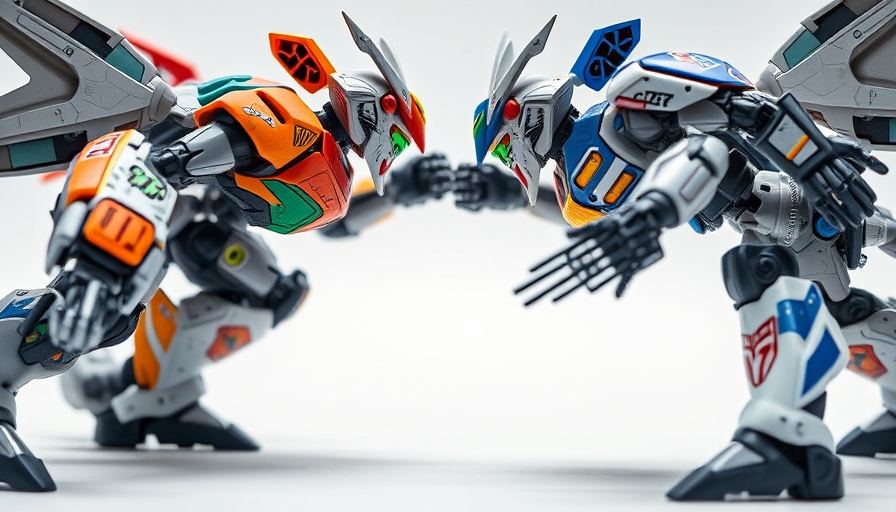
Transforming AI: How Mamba Revolutionizes Sequence Modeling Beyond Transformers
In a world where artificial intelligence (AI) is increasingly dominated by Transformers, a new contender has emerged: Mamba. Coming from the family of State Space Models (SSMs), Mamba stands out by efficiently handling long sequences of data—up to 1 million tokens—while maintaining speed and performance. This leap in technology not only challenges the Transformer architecture but also paves the way for exciting developments in various industries.
The Problem with Transformers
While Transformers are known for their ability to process complex tasks, they face significant limitations, particularly with long sequences. Each token in a Transformer can communicate with all previous tokens due to the Attention Mechanism, leading to quadratic complexity in training and memory usage. This means that as the context length expands, the time and space requirements grow exponentially—resulting in slow inference speeds and potential crashes due to memory constraints.
A New Era with Mamba
Mamba doesn't just offer a workaround for these issues; it redefines how sequence models operate. It eliminates the quadratic bottleneck by substituting the Attention Mechanism with a more streamlined approach inspired by control theory. The outcome is a model that not only scales linearly with input length but also runs up to five times faster than comparable Transformers.
Why Mamba Matters
With its unique architecture, Mamba holds promise for applications that require processing of long sequences such as in language modeling, genomics, and audio analysis. By integrating selective state management, Mamba can prioritize relevant information while discarding the rest, making it superior in handling long-range dependencies compared to both RNNs and traditional Transformers.
Looking Ahead: Mamba’s Implications for AI
If the Mamba model's capabilities can be scaled to larger datasets and tasks, it could herald a new era in AI applications, making advanced models viable on devices with limited computational power. This advancement has stunning prospects not just for chatbots, but for virtually every AI application that deals with lengthy sequences, such as automated customer service systems and even predictive analytics in finance.
The Takeaway: What Lies Ahead?
As Mamba continues to evolve and be tested in real-world applications, the implications are clear—this new architecture could reshape the landscape of AI, moving us into a post-Transformer era. With its potential to cleverly manage memory and select relevant information, Mamba is more than just a novel advance in technology; it’s a glimpse into the future of sequence modeling and AI.
Stay informed about cutting-edge developments in AI like Mamba to explore how this transition could impact you and the industries around you.
Write A Comment