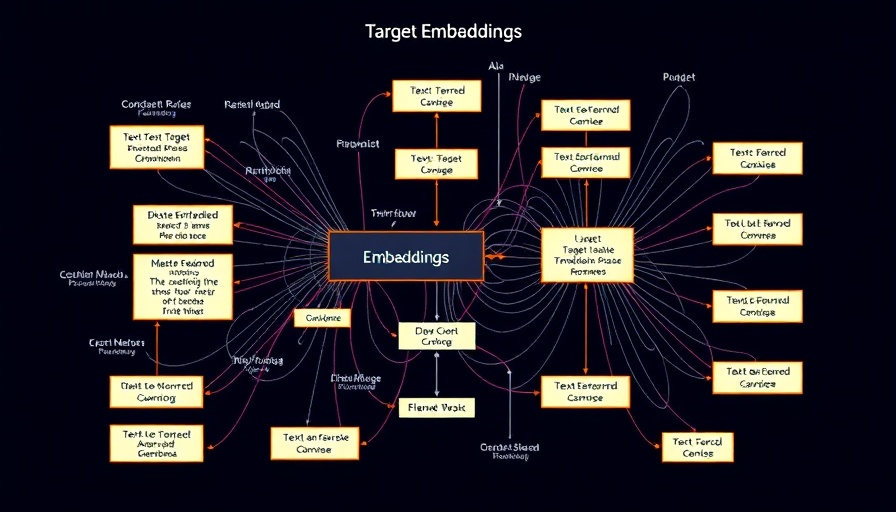
Understanding Text Embeddings: The Gateway to AI Efficiency
The world of AI has surged ahead, particularly with the integration of tools designed to provide myriad functionalities. One of the standout players in this space is Retrieval-Augmented Generation (RAG). Companies looking to adopt AI often employ RAG systems, which work by holding large libraries of documents in vector databases—collections of embeddings that serve as numerical representations of text data.
The Importance of Retrieval-Augmented Generation Systems
RAG enables AI to retrieve relevant documents from its database and generate contextual responses. This process hinges on the creation of embeddings—essentially vectors that encapsulate the meaning and similarity of the textual data stored. But there’s a twist: these embeddings often look like random strings of numbers to human eyes, containing no understandable information when viewed directly, creating a mystery around data security.
Can We Trust Text Embeddings With Sensitive Data?
With businesses relying on these vector databases to hold sensitive text data (which usually are anonymized), concerns arise regarding the potential for data recovery through hacking. Authenticity and security of this data become vital. If an attacker were to gain access to these embedding vectors, could they reconstruct the original text? Researchers recently explored whether or not this was feasible, prompting important discussions about the efficacy of text embeddings as a secure means of storing private information.
Security Enhancements in Vector Database Systems
As the landscape evolves, it’s apparent that security measures must adapt accordingly. Effective security controls are essential to safeguarding sensitive information embedded within these databases. Recommendations include implementing robust anonymization protocols before data processing, ensuring strict access controls for who can read or write to these databases, and employing encryption methods.
According to experts, simply leveraging RAG doesn’t guarantee security; proactive measures such as constant monitoring and regular audits can safeguard against unauthorized access and data breaches. Enhancing these protection mechanisms can aid developers in harnessing the powerful capabilities of AI while still maintaining a protective barrier around sensitive data.
Final Thoughts: Navigating the Future of AI with Confidence
As we advance into an era dominated by AI, understanding the depth and intricacies of this technology is crucial for ensuring data security. Therefore, while embeddings serve as a useful tool in transforming AI interactions, understanding their functionality and vulnerability will guide organizations on their journey into this brave new world. Staying informed and implementing well-researched security protocols will pave the way for safer AI technology implementation.
Write A Comment