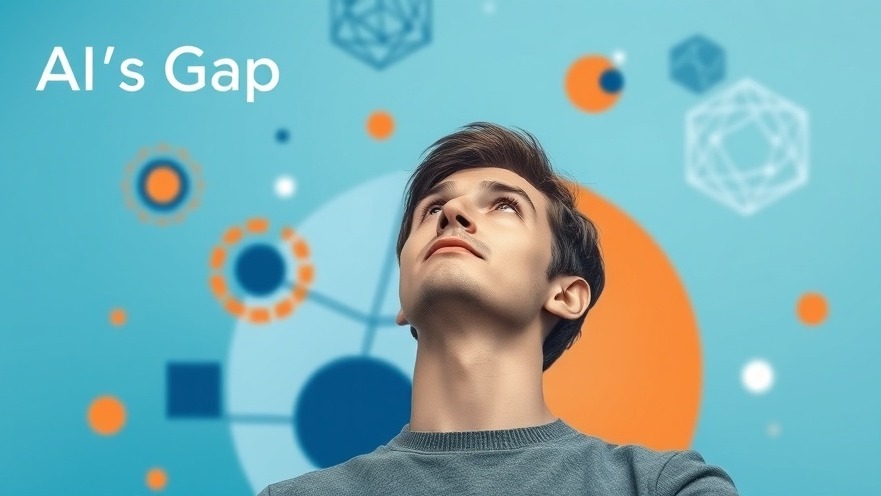
The Glaring Gap of AI Models in Spatial Reasoning and Automation
Artificial Intelligence has come a long way in achieving remarkable feats in various domains, notably with Large Language Models (LLMs) excelling in textual reasoning and mathematical benchmarks. However, one striking revelation from recent assessments is that despite their prowess in written tasks, these AI systems face severe limitations when it comes to spatial reasoning. The recent EnigmaEval benchmark has spotlighted this crucial gap, revealing the inadequacies of LLMs in problem-solving that requires spatial and geometric understanding.
Understanding AI's Shortcomings: The EnigmaEval Benchmark
The EnigmaEval benchmark was specifically designed to challenge LLMs on tasks requiring complex multimodal reasoning, particularly emphasizing spatial puzzles. While these models shine in structured text-based problems, they struggle significantly with spatial tasks that humans generally tackle with ease. The benchmark tasks illustrate how LLMs, despite being trained on vast textual datasets, lack a nuanced understanding of spatial relations and physical laws, resulting in inadequate performance.
The Reasons Behind AI's Visual Blindness
Several factors contribute to the difficulties faced by LLMs on spatial reasoning tasks:
Text-Based Training Bias: LLMs derive their knowledge primarily from text, limiting their exposure to real-world spatial dynamics, essential for developing a robust spatial intuition.
Absence of Physical Experience: Unlike humans who learn spatial relations through bodily interaction with their environment, LLMs lack sensory inputs that aid in forming accurate geometric and physical mental models.
Current Architectural Limitations: Many prevalent models, such as Transformers, excel in sequence processing but are not inherently equipped to handle spatial manipulations, exacerbating their limitations.
The Implications for Automation
The implications of LLMs’ deficiencies in spatial reasoning extend far beyond academic concerns, affecting industries reliant on AI-driven automation. Whether in robotics, manufacturing, or business processes, tasks that require spatial understanding are commonplace:
Debugging Software: Visualizing the intricate relations of code dependencies can pose challenges for AI lacking spatial comprehension.
Robotic Manipulation: The physical world’s challenges demand an understanding of movement and positioning, which current LLMs struggle to execute effectively.
Logistics and Navigation: Accomplishing efficient logistics management requires AI to process maps and navigate spaces accurately—abilities where LLMs show significant limitations.
Future Directions: Enhancing Spatial Reasoning in AI
Despite these challenges, solutions are emerging that could augment the spatial capabilities of AI systems. Approaches such as multimodal learning, where visual and spatial reasoning components are integrated into LLM frameworks, may bolster their performance. Implementing reinforcement learning in simulated environments could also enable AI to gain an embodied understanding of spatial dynamics through practice and experience.
Conclusion: Bridging the Spatial Gap
As industries become increasingly reliant on AI for complex decision-making and automation, addressing the gaps in spatial reasoning is paramount. While the EnigmaEval benchmark highlights the current limitations of LLMs, it simultaneously paves the way for innovations that could enhance these systems' cognitive flexibility. The road ahead involves a concerted effort to integrate human-like spatial reasoning capabilities into AI, ensuring that technology can fully support and transform real-world applications.
Write A Comment